Talk Summaries
February 24
Keynote speakers
Dawn Bell, Global Development Head, Novartis
Improving Drug Development Cycle Times: The Promise of AI
The purpose of this keynote is to help the community understand where the opportunities for AI are in the value-creation cycle of drug development. And importantly, to understand the challenges both technically and culturally that will need to be overcome to capitalize on these opportunities and make an impact for patients. By the end of my talk, I hope listeners are inspired to tackle problems areas that might be amenable to their expertise and to innovate new technologies to overcome the limitations of current models in addressing some of these unique challenges.
Yoshua Bengio, Scientific director, Mila
How Machine Learning Could Accelerate Drug Discovery
As robotics and biotechnology are enabling larger and larger scale screening of drug candidates, the appeal of machine learning to explore the space of molecules better and faster greatly increases. A deep network can be trained on the acquired data to map a candidate molecule to properties of interest. Reinforcement learning and generative models can be used to search for candidates which optimize some of these properties. Active learning can be used to take advantage of the uncertainty estimated for these predictions in order to close the loop between machine learning candidate generation and experimental assay results and trade-off exploration and exploitation. As the cost and success rate of drug discovery are getting worse, and as humanity is facing major threats from mutating pathogens, it becomes urgent to explore AI technologies with the potential to accelerate, reduce the costs and improve drug discovery.
Featured Presenters/Speakers
Sean Davidson and Florent Chandelier
The Promise of the DHDP: Harnessing the Power of Health Data while Protecting Privacy
The promise of the DHDP is to super-charge our ability to deliver precision medicine, which seeks to deliver the right treatment to the right patient at the right time based on that patient’s unique combination of characteristics. The DHDP’s promise to patients is that it will place protection of patient’s data and reduction of risk to the patient’s privacy at the centre of the Platform. We will establish a set of standards and apply them rigorously to all technologies operating on the DHDP. We will set up a certification service that ensures only those technologies that meet all standards are capable of accessing patient data. We will establish processes to review activity regularly across the DHDP to ensure that only to right individuals access the right data at the right time.
Ali Bashashati
Pathology in the Digital Age: Role of AI in Biomarker Discovery
AI applied to image analysis, fueled by the increased capacity of digitizing the pathology slides, offers the opportunity to objectively revisit the rich amount of information in tumour morphology. In this presentation, I will highlight the application of AI in digital pathology and how AI could be employed to accelerate translational research and biomarker discovery for cancer. I will briefly talk about the BC Translational Digital Pathology AI Initiative where we intend to integrate imaging and omics for biomarker discovery. I will then briefly highlight a few use cases that we are currently working on.
Caroline Reinhold
Radiomics as an Imaging Biomarker in Oncology: Opportunities and Challenges
Medical images contain valuable information which can be harnessed through computer-assisted analysis and prediction modeling. Radiomics as an imaging biomarker provides important information regarding temporal and spatial tumour heterogeneity. Radiomics is able to represent the complete spectrum of the disease phenotype including metastatic sites, currently not possible with tissue sampling and, therefore, could serve as a “virtual biopsy”. The clinical relevance and potential impact of radiomics in oncological imaging will be presented by using high grade serous ovarian carcinomas as a use case.
February 25
Keynote speakers
Andre Dekker, Medical Physicist & Professor of Clinical Data Science, Maastricht University & University Medical Centre, The Netherlands
The Personal Health Train – A Federated Health Data Infrastructure
Artificial intelligence, big data, machine learning and data science are expected to have a major impact on day-to-day cancer practice with the first AI products already available to the cancer community. But to be successful, these innovations need lots of data to be developed and validated and getting access to sufficient data is hampered by administrative, political, ethical and technical barriers. Since 2008 Maastricht University has embarked on an R&D program to build a global federated, privacy-preserving, FAIR data infrastructure called the Personal Health Train. The rationale, challenges and first results of this infrastructure will be discussed.
David Jaffray, Senior Vice-President and Chief Technology Officer, University of Texas MD Anderson Cancer Center
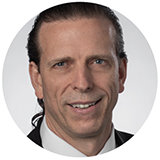
Data Microeconomics – Supply and Demand
The development of machine learning has further accelerated our capacity to consume data. While the technology has had a dramatic impact on our ability to mimic, predict, and extract insights, it is also putting remarkable pressure on the processes by which data is generated, governed, and consumed. The connection between supply and demand in the data economy is now starting to form and sets us on the long journey toward supply and demand equilibrium. Progress on this journey will (i) reinforce the importance of data governance within and between individuals and organizations and (ii) drive the development of data governance technologies (DGTs) to allow the data economy to operate fairly and efficiently.
Featured Presenters/Speakers
Martin Lepage & Kafi Kam
Requirements for a performant AI platform
Access to a performant artificial intelligence (AI) platform to analyse sets of images has the potential to multiply the usefulness of medical imaging in the detection, the diagnosis, the prediction of treatment response and for a personalized treatment follow-up. For this, several critical functions must be as user-friendly as possible, including the possibility for multi-centric studies. Such requirements will be presented.
Lillian Siu & Benjamin Haibe-Kains
Use Case: Integrating Multi-Omic Data in MOHCCN to Identify Predictive Biomarkers in Immunotherapy
In this use case, Marathon of Hope Cancer Centre Network (MOHCCN) cohorts which have clinical annotation along with multi-omic data including genomics, transcriptomics, immunoprofiling, radiomic, microbiome and other technologies will be discussed, using the Princess Margaret Cancer Centre INSPIRE study (NCT02644369) as an example. MOHCCN and the DHDP provide the framework for data in these information-rich cohorts to be shared. The application of AI/machine learning models to explore, evaluate and validate potential predictive biomarkers of immune sensitivity or resistance using these integrated data sets increases the scientific value and computational power of any single cohorts alone.
Christy Holtby and Randy Goebel
Road Map to Alberta
An introduction to the Alberta Machine Intelligence Institute & the Alberta ecosystem. This session will highlight lessons learned at the intersection of AI in Health with a particular focus on the collaboration between Amii and the University of Alberta’s Precision Health Signature Area. Learn our approach to collaboration, where we’ve been, where we are poised to go - we hope you will join us in this journey.